DUMMY'S GUIDE TO BELIEF NETWORKS
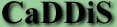
The Bayesian
Belief Network methodology provides a mathematical technique which
can be used to assess quantitatively the effect which different pieces
of information will have on our belief in a variety of different scenarios.
In doing this, it merely automates and makes precise an activity which
we all constantly carry out. The following example will illustrate the
concept:
You wish to guess the nationality of a tourist sitting at a neighbouring
table in a cafe in Glasgow. What nationality would you suspect if realised
that he was speaking in Finnish? How would the conclusion differ if instead
you heard him speaking in French?
Finnish is a language which has no relation to most of the other languages
of Europe. It is correspondingly more difficult for non-Finns to learn
the language than to learn other Indo- European languages. This is reflected
in the statement that
Prob(He can speak Finnish | He is not Finnish) is close to zero.
This is a conditional
probability. It tells us about the chances of something happening conditional
on something else being true.
However, it is true that
Prob(He can speak Finnish | He is Finnish) will be close to one (since
most citizens of Finland can speak their national language).
It is intuitively obvious that if these two probability statements
are true then our observation that the tourist can speak Finnish is strong
evidence that he is, indeed, a Finn.
By contrast, French is an international language which is taught as
a second language in many other countries. In addition, both Belgium and
Switzerland contain sizeable populations whose mother-tongue is French.
Hence it follows that
Prob(He can speak French | He is not French) may be relatively high.
Hence, although
Prob(He can speak French | He is French) will be close to one, it is
intuitively clear that since speaking French is not such a distinctive
mark of nationality as is speaking Finnish, an observation that the tourist
is speaking French is not particularly strong proof that he is French.
Life rarely involves the isolated consideration of single pieces of
information. We might hear the tourist speaking English to the waiter,
and recognise that he is speaking with a Scandinavian accent. If we had
previously heard him speak in Finnish, this would add little to our knowledge.
However, if we had earlier heard him speaking in French, we might adjust
our ideas to conclude that he is probably a Scandinavian who happens to
speak excellent French (though he might be a Frenchman who was taught English
by a Swede!)
Examples such as the above can get very complicated! This merely illustrates
the point that the human brain becomes overloaded when asked to evaluate
large quantities of conditional information. In particular, we tend to
give too much weight to new information which confirms earlier evidence,
even where the new information follows as a consequence of the earlier
evidence. e.g. If the tourist had earlier spoken in Finnish, we was very
likely to also have a Scandinavian accent. our observation of this fact
does not add much to our earlier knowledge of his nationality.
The Bayesian
Belief Network takes a number of pieces of evidence and consistently
evaluates their joint significance to determine the relative plausibility
of different hypotheses. It does this using a variant of Bayes's
Rule (a mathematical rule to relate conditional
probabilities) acting on a network of relationship between different
observations and hypotheses. The numbers which the Bayesian network generates
are known as beliefs because they describe the belief which we might have
in different hypotheses (such as nationality of tourist, or disease of
cow) given the observation of particular pieces of evidence (such as language
spoken or clinical sign observed).